4 min to read
Statistics - Inferential Statistics - Type of statistical study
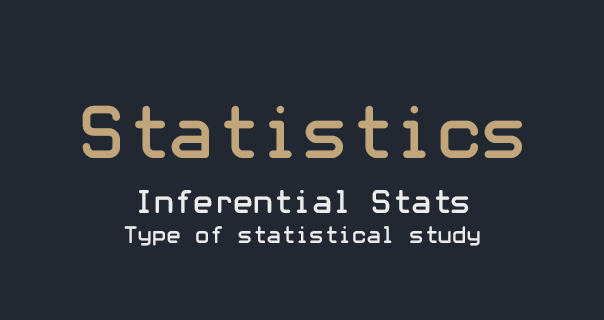
This article is a part of the Statistics - 101 series, you can access the full version of the series here:
-
Foundation
-
Descriptive Statistics
-
Inferential Statistics
- Type of statistical study (you are here!)
- Probability and distribution
- Hypothesis testing
- Estimation
- Regression
Welcome to the fourth article of the Statistics 101 series. We have covered different ways to understand our data using descriptive statistics. But there is another side of Statistics that we have not touched upon, which is Inferential Statistics.
Inferential Statistics is clearly explained in part 1 of Statistics Foundation article a while ago. You can access it here. Briefly speaking, inferential statistics allows us to generalize beyond collections of actual observations, which can also be understood as to get a conclusion for the whole population using a sample of such population.
To achieve such result, we need to apply statistical study, which will be discussed in this article.
- 1. Two Types of Statistical Study
- 1.1. Observational Study
- 1.2. Experimental Study
- 2. How to achieve a sample for statistical study
1. Two Types of Statistical Study
1.1. Observational Study
The name says its own, right? In observational study, we simple observe the behaviors of the subject of interest, without interfering with anything.
Observational study is a study that focuses on detecting relationships between variables no manipulated by the investigator.
Example: A researcher might collect paired measures of income per capita level and crime rate for each individual in some group. By conducting statistical analysis on these data, we are conducting observational study.
Advantages:
- Easy to conduct, since we only need to collect data
Disadvantages:
-
We can only conclude an correlation between the variables, not a cause-effect relationship.
In the last example, this disadvantage might not be clear, so let’s take another example. Imagine we have data on number of sunglasses sold and number of ice-cream sold. The statistical analysis we conduct on the data reveals a positive linear relationship between sunglasses and ice-cream (ice-cream sales increases, sunglasses sales increases and vice versa).
However, we cannot conclude that the rise in ice-cream sales lead to the rise in sunglasses sales (a cause effect relationship, cause: rise in ice-cream sales, effect: rise in sunglasses sales). The two effects might be due to another cause, maybe because of the hot weather. So due to the hot weather, both sales increase.
This is correlation, and it is the only conclusion that we can obtain from an observational analysis
1.2. Experimental Study
To mitigate the drawback of observational study, experimental study is usually adopted.
Experimental study is a study in which conditions are controlled and manipulated by the experimenter
Example: To see the effect of smoking on health, researchers conduct a 5 year experiment on two groups of volunteer, one group is allowed to smoke and one is not.
Advantages:
- Can reveal cause-effect relationship
Disadvantages:
- Resource-consuming
2. How to achieve a sample for statistical study
2.1. Observational - Random sampling
Whenever possible, a sample should be randomly selected from a population in order to increase the likelihood that the sample accurately represents the population.
Random sampling is a procedure designed to ensure that each potential observation in the population has an equal chance of being selected in a survey.
Random sampling introduce a non-bias data to the analysis, which is required for a valid conclusion of the average effect to be made.
Random sampling can be very difficult when a population lacks structure (e.g., all persons currently in psychotherapy) or specific boundaries (e.g., all volunteers who could conceivably participate in an experiment). In this case, a random sample becomes an ideal that can only be approximated - always with an effort to remove obvious biases that might cause the sample to misrepresent the population
2.2. Experiments - Random assignments
In experimental study, the average effect is usually not of interest. For example, Estimating the average anxiety score for all college students probably would not generate much interest. Instead, we might be interested in determining whether relaxation training causes, on average, a reduction in anxiety scores between two groups of otherwise similar college students.
Random assignment signifies that each person has an equal chance of being assigned to any group in an experiment
Advantages:
Not only random assignment minimizes any biases that might favor one group or another, it also serves as a basis for estimating the role of variability in any observed result, allowing us to determine whether such difference is real or mere transitory.
Comments